Product Analytics has emerged as a critical tool for understanding user behavior and driving product success. This playbook serves as a comprehensive guide to help businesses harness the power of Product Analytics to improve product performance, enhance user experience, and achieve long-term growth. From defining objectives to implementing the right tools and conducting in-depth analysis, this playbook covers key steps and best practices for leveraging Product Analytics effectively.
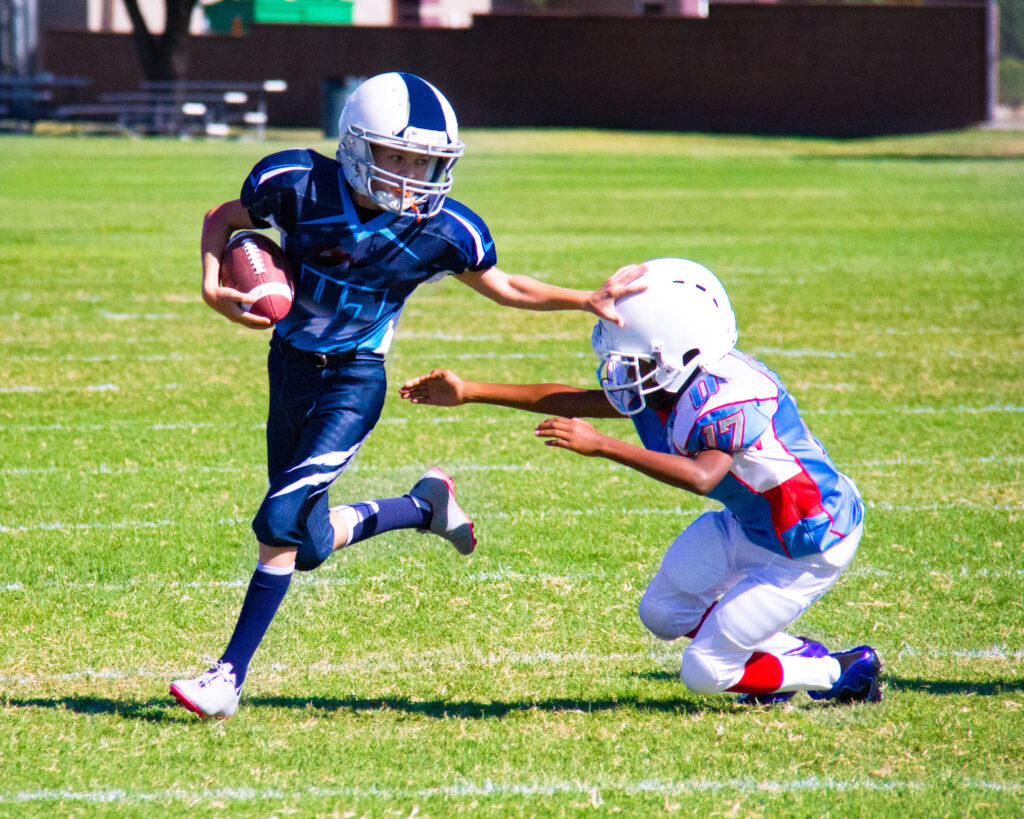
- Define Clear Objectives:
The first step in any Product Analytics strategy is to define clear and measurable objectives. Understanding what you aim to achieve will shape the entire analytics approach. Common objectives include:
a. Enhancing User Engagement: Increasing user engagement metrics, such as time spent in the product, feature adoption, and frequency of use.
b. Improving User Onboarding: Reducing friction during the onboarding process to boost the number of active users.
c. Optimizing Conversion Rates: Identifying bottlenecks in the conversion funnel and optimizing it to increase the rate of successful conversions.
d. Reducing Churn: Analyzing user drop-off points and addressing pain points to minimize churn rate.
e. Personalization: Tailoring user experiences based on segmentation and user preferences to drive user satisfaction.
- Identify Key Performance Indicators (KPIs):
Once objectives are set, determine the Key Performance Indicators (KPIs) that align with these goals. KPIs provide quantifiable metrics to measure success. Some common Product Analytics KPIs include:
a. Adoption Rate: The percentage of users who have successfully onboarded and started using the product.
b. Retention Rate: The percentage of users who continue using the product over a specified period.
c. Engagement Metrics: Time spent in the product, feature usage, and session duration.
d. Churn Rate: The rate at which users stop using the product.
e. Net Promoter Score (NPS): A metric used to assess customer loyalty and satisfaction.
- Select Appropriate Product Analytics Tools:
Choosing the right analytics tools is crucial for effectively collecting, processing, and analyzing product data. Some popular Product Analytics tools include:
a. Mixpanel: An event-based analytics tool that enables granular tracking of user interactions.
b. Amplitude: Specializing in user behavior analytics to identify patterns and trends.
c. Google Analytics for Apps: A comprehensive analytics solution for mobile applications.
d. Heap Analytics: Automatically captures all user interactions without requiring prior event setup.
e. Kissmetrics: Focuses on customer journey analysis and user segmentation.
- Data Collection and Integration:
With the chosen analytics tools in place, ensuring seamless data collection from various sources is essential. This may include mobile apps, websites, and other product touchpoints. Data integration is crucial for a holistic view of user behavior and interactions across different platforms.
a. Event Tracking: Set up event tracking to capture user actions, such as clicks, searches, and form submissions.
b. User Identification: Implement user identification methods to track individual user behavior across sessions and devices.
c. Data Warehousing: Integrate Product Analytics data with other relevant data sources, such as CRM systems or customer support tools.
d. Data Quality Assurance: Regularly audit data quality to ensure accuracy and consistency.
- User Segmentation:
User segmentation enables personalized experiences, allowing businesses to cater to specific user groups with tailored content and features. Segmentation can be based on demographics, behavior, geography, or other relevant criteria.
a. Cohort Analysis: Group users based on similar characteristics or behavior patterns during a specific time frame.
b. Behavior-Based Segmentation: Segment users based on their interactions with specific features or actions.
c. Demographic Segmentation: Categorize users based on age, gender, location, or other demographic factors.
d. Persona Creation: Develop user personas to understand target audiences and their needs better.
- Conduct A/B Testing:
A/B testing is a powerful technique that helps compare different variations of a product or feature to identify the most effective one. Businesses can make data-backed decisions on design changes or feature implementations by conducting controlled experiments.
a. Hypothesis Formulation: Define clear hypotheses before conducting A/B tests to ensure the tests address specific objectives.
b. Randomized Samples: Ensure randomization of users into test groups to avoid bias.
c. Analyze Results: Analyze the data to determine which variation performs better based on predefined KPIs.
d. Iterative Testing: Continuously iterate and refine A/B tests to optimize results.
- Funnel Analysis:
Funnel analysis involves tracking user journeys through predefined sequences of actions to identify drop-off points and areas for improvement. Understanding where users drop out of the conversion funnel is crucial to optimizing conversion rates.
a. Define Funnels: Create funnels based on user actions leading to a specific conversion goal.
b. Analyze Funnel Steps: Identify steps with significant drop-off rates and investigate potential causes.
c. Optimization Strategies: Implement changes to reduce friction and improve conversion rates at critical funnel stages.
- User Feedback and Qualitative Analysis:
While quantitative data is essential, qualitative user feedback provides valuable insights into pain points and user preferences.
a. Surveys and Interviews: Conduct user surveys and interviews to gather feedback on user experiences and pain points.
b. Customer Support Analysis: Analyze customer support data to identify common issues and user concerns.
c. User Testing: Conduct usability tests with real users to observe their interactions and identify usability issues.
- Data Visualization and Reporting:
Effectively communicating insights to stakeholders is vital for driving data-informed decision-making within the organization.
a. Dashboards: Build visual dashboards that present key metrics and KPIs in real time.
b. Executive Reports: Create concise and insightful reports for executive teams to aid strategic decision-making.
c. Data Storytelling: Use data visualization techniques to tell compelling stories and present actionable insights.
Product Analytics is an iterative process that requires continuous monitoring and improvement.
a. Review and Iterate: Regularly review analytics performance and iterate on strategies based on feedback and outcomes.
b. Cross-functional collaboration: Foster collaboration between product teams, marketing, customer support, and analytics teams for a holistic approach.
c. Stay Updated: Keep up with the latest trends and advancements in Product Analytics to leverage new tools and methodologies.
Conclusion:
The Product Analytics Playbook serves as a comprehensive guide for businesses seeking to harness the power of data to drive product success. By defining clear objectives, selecting appropriate tools, and conducting in-depth analysis, businesses can unlock valuable insights to optimize user experiences, increase engagement, and achieve long-term growth. Embracing a data-driven approach and continuously improving based on insights will position organizations for sustained success in the dynamic digital landscape.